Research
I work in the Inan Research Lab at Georgia Tech. We specialize in wearable health solutions such as noninvasive cardiovascular and joint monitoring, and frequently use machine learning techniques in our search for clinically relevant outcomes.
My work focuses on noninvasive cardiovascular and cardiopulmonary monitoring, specifically for the purpose of long-term monitoring and assisting in triage and resuscitation following traumatic injury.
Our bodies are able to compensate (up to a certain level) when faced with an injury or other condition that pushes us out of homeostasis. I’m working on quantifying how much more a person can compensate in such a situation to give actionable insight to the individual and other decision makers such as healthcare or emergency response teams.
The four main noninvasive signals I work with are the electrocardiogram (ECG), seismocardiogram (SCG), photoplethysmogram (PPG), and ballistocardiogram (BCG).
Here’s a quick breakdown of these signals:
- The ECG is measured with electrodes and monitors the electrical activity of the heart
- The SCG measures the local vibrations of the chest wall due to heart, lung and blood movement with an accelerometer and/or gyroscope. In this sense you are able to measure the ‘mechanical’ function of the heart.
- The PPG uses an LED/photodiode combo to measure pulsatile changes in light absorption in the underlying tissue due to blood flow. This can give you quite a bit of information about the vasculature at the chosen distal location.
- The BCG measures whole body movement due to blood being ejected out of the heart, typically with load cells in various formations.
These four signals are frequently used to estimate cardiac timing intervals such as the pre-ejection period, left ventricular ejection time, and pulse transit time. These cardiac timing intervals are very clinically relevant. Some ratios, such as PEP/LVET, also contain a lot of information. PEP/LVET is a known indicator of left ventricular performance.
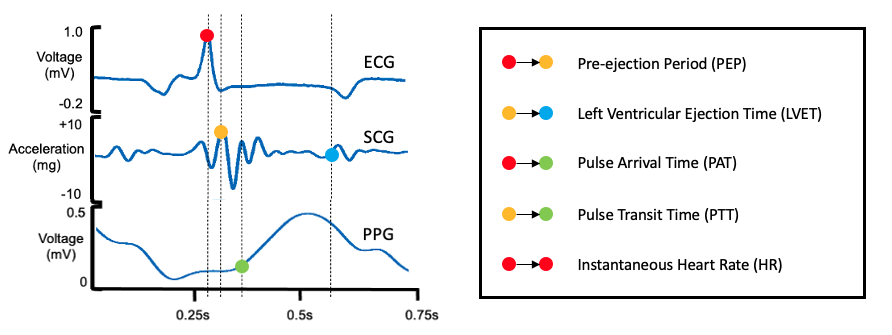
Of course, other features from these signals can also be extracted for use in machine learning models to make different predictions.
Note: I’m slowly building up this page, but if you’d like more specifics about what I do, check out my publications.
My Research in the news: